Enhancing EV Performance Tuning and Customization Techniques
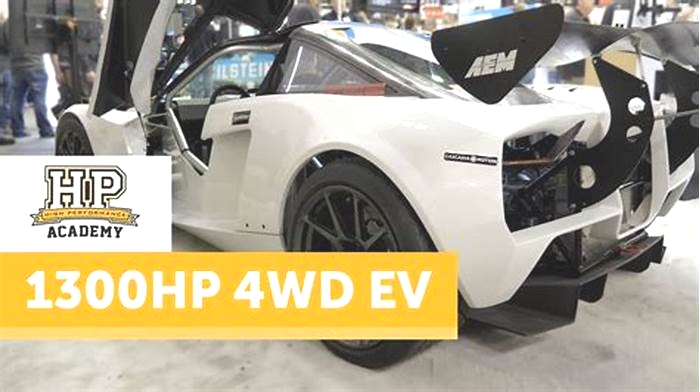
Customizing Large Language Models: A Comprehensive Guide
In an age where artificial intelligence impacts almost every aspect of our digital lives, have we fully unlocked the potential of Large Language Models (LLMs)? Are we harnessing their capabilities to the fullest, ensuring that these sophisticated tools are finely tuned to address our unique challenges and requirements? The journey of customizing Large Language Models represents a pivotal shift towards creating more intelligent, responsive, and contextually aware Generative AI systems that cater to a broad spectrum of applicationsfrom simplifying customer service interactions to driving innovation in fields like healthcare and finance.
Large Language Models, with their profound ability to understand and generate human-like text, stand at the forefront of the AI revolution. But to truly leverage their power, customization is key. This involves fine-tuning pre-trained models on specialized datasets, adjusting model parameters, and employing techniques like prompt engineering to enhance model performance for specific tasks. Customizing LLMs allows us to create highly specialized tools capable of understanding the nuances of language in various domains, making AI systems more effective and efficient.
In this comprehensive guide, we delve into the world of Large Language Modelsexploring their foundational principles, the importance of customization, and the methodologies that enable us to tailor these models to our specific needs.
Understanding Large Language Models
At the heart of modern artificial intelligence systems lies a groundbreaking technology known as Large Language Models. These advanced models, powered by deep learning algorithms and massive datasets, have revolutionized the field of natural language processing (NLP), enabling machines to understand, interpret, and generate human-like text with remarkable accuracy. But what makes LLMs so pivotal in the AI landscape, and how can we harness their full potential through customization?
The Evolution of LLMs: From RNNs to Transformers
The evolution of LLMs from simpler models like RNNs to more complex and efficient architectures like transformers marks a significant advancement in the field of machine learning. Transformers, known for their self-attention mechanisms, have become particularly influential, enabling LLMs to process and generate language with an unprecedented level of coherence and contextual relevance.
Foundation Models: The Building Blocks of Custom LLMs
At the heart of customizing LLMs lie foundation modelspre-trained on vast datasets, these models serve as the starting point for further customization. They are designed to grasp a broad range of concepts and language patterns, providing a robust base from which to fine-tune or adapt the model for more specialized tasks.
Exploring Popular Foundation Models: Llama 2, BLOOM, Falcon, MPT
Several community-built foundation models, such as Llama 2, BLOOM, Falcon, and MPT, have gained popularity for their effectiveness and versatility. Llama 2, in particular, offers an impressive example of a model that has been optimized for various tasks, including chat, thanks to its training on an extensive dataset and enrichment with human annotations.
These models represent the forefront of efforts to harness the power of LLMs for a wide array of applications, showcasing the potential of custom LLMs to transform industries by generating human-like text, facilitating retrieval augmented generation, and driving the development of contextually aware AI systems.
The Process of Customizing LLMs
Customizing LLMs is a sophisticated process that bridges the gap between generic AI capabilities and specialized task performance. This process involves a series of steps designed to refine and adapt pre-trained models to cater to specific needs, enhancing their ability to understand and generate language with greater accuracy and relevance.
The journey of customization begins with data collection and preprocessing, where relevant datasets are curated and prepared to align closely with the target task. This foundational step ensures that the model is trained on high-quality, relevant information, setting the stage for effective learning.
Following this, the selection of the foundation model is crucial. Choosing the right pre-trained model involves considering the models size, training data, and architectural design, all of which significantly impact the customizations success. With models like Llama 2 offering versatile starting points, the choice hinges on the balance between computational efficiency and task-specific performance.
Model customization itself is where the magic happens. Through fine-tuning or employing more nuanced techniques like prompt engineering or Parameter-Efficient Fine-Tuning (PEFT), described more thoroughly in the next section, developers can significantly alter the models behavior to excel at the desired tasks. This step is both an art and a science, requiring deep knowledge of the models architecture, the specific domain, and the ultimate goal of the customization.
Deployment and real-world application mark the culmination of the customization process, where the adapted model is integrated into operational processes, applications, or services. This phase involves not just technical implementation but also rigorous testing to ensure the model performs as expected in its intended environment.
Finally, monitoring, iteration, and feedback are vital for maintaining and improving the models performance over time. As language evolves and new data becomes available, continuous updates and adjustments ensure that the model remains effective and relevant.
This iterative process of customizing LLMs highlights the intricate balance between machine learning expertise, domain-specific knowledge, and ongoing engagement with the models outputs. Its a journey that transforms generic LLMs into specialized tools capable of driving innovation and efficiency across a broad range of applications.
Techniques for Customizing LLMs
Customizing Large Language Models for specific applications or tasks is a pivotal aspect of deploying these models effectively in various domains. This customization tailors the models outputs to align with the desired context, significantly improving its utility and efficiency. Here, we delve into several key techniques for customizing LLMs, highlighting their relevance and application in enhancing model performance for specialized tasks.
Fine Tuning: Tailoring Pre-Trained Models for Specific Tasks
Fine tuning is a widely adopted method for customizing LLMs, involving the adjustment of a pre-trained models parameters to optimize it for a particular task. This process utilizes task-specific training data to refine the model, enabling it to generate more accurate and contextually relevant outputs. The essence of fine tuning lies in its ability to leverage the broad knowledge base of a pre-trained model, such as Llama 2, and focus its capabilities on the nuances of a specific domain or task. By training on a dataset that reflects the target task, the models performance can be significantly enhanced, making it a powerful tool for a wide range of applications.
Retrieval Augmented Generation: Enhancing LLMs with External Data
Retrieval Augmented Generation (RAG) is a technique that combines the generative capabilities of LLMs with the retrieval of relevant information from external data sources. This method allows the model to access up-to-date information or domain-specific knowledge that wasnt included in its initial training data, greatly expanding its utility and accuracy.
RAG operates by querying a database or knowledge base in real-time, incorporating the retrieved data into the models generation process. This approach is particularly useful for applications requiring the model to provide current information or specialized knowledge beyond its original training corpus.
Prompt Engineering: Guiding LLMs to Desired Outputs
Prompt engineering is a technique that involves crafting input prompts to guide the model towards generating specific types of responses. This method leverages the models pre-existing knowledge and capabilities without the need for extensive retraining. By carefully designing prompts, developers can effectively instruct the model to apply its learned knowledge in a way that aligns with the desired output. Prompt engineering is especially valuable for customizing models for unique or nuanced applications, enabling a high degree of flexibility and control over the models outputs.
Parameter-Efficient Fine-Tuning Methods: P-tuning and LoRA
Parameter-Efficient Fine-Tuning methods, such as P-tuning and Low-Rank Adaptation (LoRA), offer strategies for customizing LLMs without the computational overhead of traditional fine tuning. P-tuning introduces trainable parameters (or prompts) that are optimized to guide the models generation process for specific tasks, without altering the underlying model weights. LoRA, on the other hand, focuses on adjusting a small subset of the models parameters through low-rank matrix factorization, enabling targeted customization with minimal computational resources. These PEFT methods provide efficient pathways to customizing LLMs, making them accessible for a broader range of applications and operational contexts.
Each of these techniques offers a unique approach to customizing LLMs, from the comprehensive model-wide adjustments of fine tuning to the efficient and targeted modifications enabled by PEFT methods. By selecting and applying the most appropriate customization technique, developers can create highly specialized and contextually aware AI systems, driving innovation and efficiency across a broad range of domains.
Implementing Custom LLMs: A Step-by-Step Guide
Data Collection and Preprocessing for Custom Models
The foundation of any custom LLM is the data its trained on. Collecting a diverse and comprehensive dataset relevant to your specific task is crucial. This dataset should cover the breadth of language, terminologies, and contexts the model is expected to understand and generate. After collection, preprocessing the data is essential to make it usable for training. Preprocessing steps may include cleaning (removing irrelevant or corrupt data), tokenization (breaking text into manageable pieces, such as words or subwords), and normalization (standardizing text format). These steps help in reducing noise and improving the models ability to learn from the data.
Choosing the Right Foundation Model and Customization Technique
The next step is to select an appropriate foundation model. Foundation models like Llama 2, BLOOM, or GPT variants provide a solid starting point due to their broad initial training across various domains. The choice of model should consider the models architecture, the size (number of parameters), and its training datas diversity and scope. After selecting a foundation model, the customization technique must be determined. Techniques such as fine tuning, retrieval augmented generation, or prompt engineering can be applied based on the complexity of the task and the desired model performance.
Selecting the Right Model Size for Your Use Case
Model size, typically measured in the number of parameters, directly impacts the models capabilities and resource requirements. Larger models can generally capture more complex patterns and provide more accurate outputs but at the cost of increased computational resources for training and inference. Therefore, selecting a model size should balance the desired accuracy and the available computational resources. Smaller models may suffice for less complex tasks or when computational resources are limited, while more complex tasks might benefit from the capabilities of larger models. How to select the right size? Well, start out with a robust one, check the benchmarks, scale it down to a model with a lower amount of parameters, and check the output against benchmarks. It is all a question that comes down to a specific use case you might have.
The Iterative Process of Model Training and Fine-Tuning
Customizing an LLM is an iterative process that involves training the model on your specific dataset, evaluating its performance, and fine-tuning the parameters to optimize for the task. This process might include:
- Initial training: Begin with training or fine-tuning the selected foundation model on your curated dataset. This step adapts the model to the domain-specific language and tasks.
- Evaluation: After the initial training phase, evaluate the models performance using a separate validation dataset. This evaluation should focus on metrics relevant to the task, such as accuracy, fluency, or domain-specific benchmarks.
- Fine-tuning: Based on the evaluation, adjust the training parameters, data, or both, and fine-tune the model to improve its performance. This might involve adjusting learning rates, adding more training data, or employing different customization techniques.
- Iterative refinement: The training and fine-tuning process is typically iterative. Multiple rounds of evaluation and adjustment may be necessary to achieve the desired model performance.
Customization in Action: Use Cases and Applications
The customization of Large Language Models and the implementation of Retrieval Augmented Generation systems find critical applications across a wide range of industries. These advanced AI techniques can significantly enhance operational efficiency, user experience, and the generation of valuable insights from data. Below are several key industry use cases where LLM customization or RAG system implementation might be particularly beneficial:
- Healthcare: Custom LLMs can interpret medical literature, patient records, and research data to support diagnosis, treatment recommendations, and patient interaction, ensuring responses are tailored to the specific medical context.
- Legal sector: Tailored LLMs can assist in analyzing legal documents, contracts, and legislation, providing summaries, identifying relevant precedents, and drafting legal advice, all customized to the nuances of legal language and jurisdictions.
- Finance and banking: Customized models can enhance customer service through personalized financial advice, fraud detection analysis, and automated regulatory compliance checks, adapting to the specific financial products and regulations.
- Customer support: By integrating RAG systems, companies can provide highly accurate, context-aware customer support, drawing from a broad knowledge base to deliver personalized solutions.
- Education: Tailored educational content creation, personalized learning experiences, and automated grading can be achieved with customized LLMs, adapting to individual learning styles and curriculum requirements.
- Content creation and media: Custom LLMs can generate creative content, such as articles, marketing copy, and scripts, tailored to specific audiences, styles, and brand guidelines.
- eCommerce and retail: Personalized product descriptions, customer interaction, and recommendation systems can be enhanced using LLMs customized to understand and generate content aligned with current trends and inventory.
Challenges and Considerations in LLM Customization
Developing a custom LLM for specific tasks or industries presents a complex set of challenges and considerations that must be addressed to ensure the success and effectiveness of the customized model.
Balancing Computational Resources and Model Capabilities
One of the primary challenges, when you try to customize LLMs, involves finding the right balance between the computational resources available and the capabilities required from the model. Large models require significant computational power for both training and inference, which can be a limiting factor for many organizations. Customization, especially through methods like fine-tuning and retrieval augmented generation, can demand even more resources. Innovations in efficient training methods and model architectures are essential to making LLM customization more accessible.
Ensuring Up-to-Date Information and Reducing Model Hallucinations
Another critical challenge is ensuring that the model operates with the most current information, especially in rapidly evolving fields. LLMs, by nature, are trained on vast datasets that may quickly become outdated. Techniques such as retrieval augmented generation can help by incorporating real-time data into the models responses, but they require sophisticated implementation to ensure accuracy. Additionally, reducing the occurrence of hallucinations, or instances where the model generates plausible but incorrect or nonsensical information, is crucial for maintaining trust in the models outputs.
The Importance of Domain Expertise in Model Customization
Domain expertise is invaluable in the customization process, from initial training data selection and preparation through to fine-tuning and validation of the model. Experts not only contribute domain-specific knowledge that can guide the customization process but also play a crucial role in evaluating the models outputs for accuracy and relevance. Their insights help in adjusting the models parameters and training process to better align with the specific requirements of the task or industry.
The Potential of Custom LLMs in Transforming Operational Processes
Custom LLMs offer the ability to automate and optimize a wide range of tasks, from customer service and support to content creation and analysis. By understanding and generating human-like text, these models can perform complex tasks that previously required human intervention, significantly reducing the time and resources needed while increasing output quality. Furthermore, the flexibility and adaptability of custom LLMs allow for continuous improvement and refinement of operational processes, leading to ongoing innovation and growth.
From healthcare and finance to education and entertainment, the potential applications of custom LLMs are vast and varied. In healthcare, for example, custom LLMs can assist with diagnostics, patient care, and medical research. In finance, they can enhance fraud detection, risk analysis, and customer service. The adaptability of LLMs to specific tasks and domains underscores their transformative potential across all sectors.
As we stand on the brink of this transformative potential, the expertise and experience of AI specialists become increasingly valuable. nexocodes team of AI experts is at the forefront of custom LLM development and implementation. We are committed to unlocking the full potential of these technologies to revolutionize operational processes in any industry. Our deep understanding of machine learning, natural language processing, and data processing allows us to tailor LLMs to meet the unique challenges and opportunities of your business.
Are you ready to explore the transformative potential of custom LLMs for your organization? Contact nexocodes AI experts today. Let us help you harness the power of custom LLMs to drive efficiency, innovation, and growth in your operational processes.